Blockchain security isn't optional.
Protect your smart contracts and DeFi protocols with Three Sigma, a trusted security partner in blockchain audits, smart contract vulnerability assessments, and Web3 security.
Get a Quote Today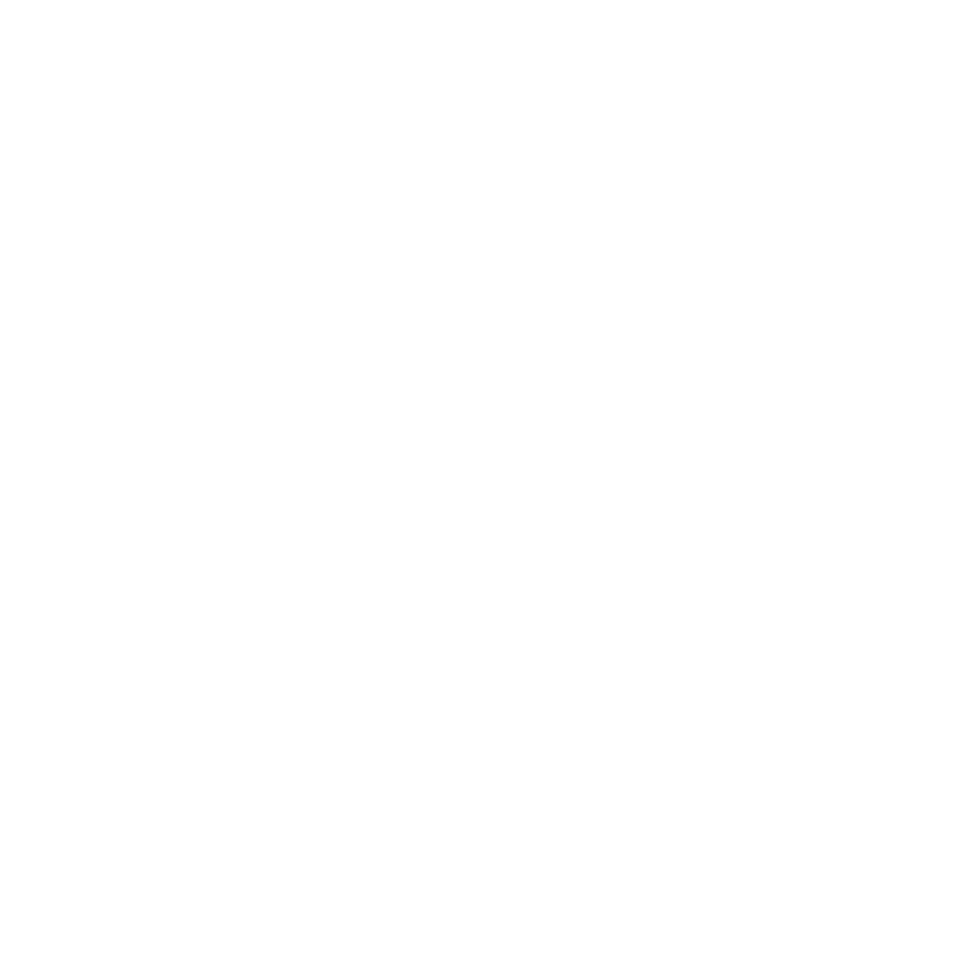
Introduction
In the web3 ecosystem, it is ideal to measure risk automatically and in a decentralised manner, relying solely on on-chain information due to its efficiency, transparency, and trustlessness advantages. However, developing insurance mechanisms for the DeFi sector presents significant challenges and diverges from conventional capital market practises.
This second article will look at the inner workings of the pricing models and risk used by the different protocols.
If you missed the first part of the series, which provides a market overview of DeFi Insurance as well as an explanation of how they work, you can find it here.
DeFi Insurance Pricing & Risk Models
Traditional insurance companies’ business strategies rely on diversifying risk, and these businesses usually generate revenue in two ways: charging premiums and reinvesting them. Each policy has a premium based on its risk, and after it is sold, the insurance firm traditionally invests it in safe short-term interest-bearing assets to avoid insolvency in case there are payouts. Generally, if a company efficiently prices its risk, it should generate more income in premiums than it spends on conditional payouts.
One critical aspect of DeFi is the relationship between yield and risk, where higher yields are often associated with greater exposure to risk. DeFi yields are significantly higher than those found in traditional finance, reflecting the unique nature of this nascent industry. However, the inherent complexity, novelty, and immutability of DeFi also present significant risks, making it essential to consider insurance solutions to mitigate potential losses. In the following section, we will explore different risk and price approaches in DeFi Insurance protocols and highlight the differences.
Exploring Different Risk & Price Approaches
In this section, we will delve into the various methodologies and strategies used to assess risk and determine pricing in the decentralised insurance space.
Nexus Mutual
Nexus Mutual was the first DeFi insurance protocol and therefore introduced the first model to price a decentralised cover product. In their V1, they utilised a market-based pricing mechanism that combined a base risk calculation with the TVL of staked cover providers to determine the premium off-chain.
A more significant amount of staked NXM indicates that after risk assessment, cover providers feel comfortable depositing funds in that pool, resulting in a lower risk cost and lower premium for that pool. A strong assumption is made here, which is the basis for the whole pricing system: cover providers stake more money in protocols they consider safer and believe they will not have to pay out. Due to the close correlation between incentives for capital providers to stake in specific pools and the expected APY (Annual Percentage Yield), their risk assessment judgement can be obscured or influenced. Finally, users should perform due diligence on each protocol to be able to properly measure risk, but most lack the technical expertise required to do it. Therefore, the value staked may not accurately reflect a protocol's risk.
It is also important to notice that there are capacity limits on the amount of cover that is offered for specific risks, protecting the protocol from being too exposed to risks. There is a Specific Risk Limit that varies with the amount of staking on a particular risk and a Global Capacity based on the total resources of the mutual. The Specific Risk Limit is calculated as the capacity factor times the net staked NXM (defined above). Governance can update these capacity factors, but setting a cap reduces the demand for cover.
Protocols started to develop new pricing mechanisms that enhanced their existing pricing models to better adapt to DeFi. In V2, Nexus Mutual replaced their off-chain pricing model with a dynamic pricing model that operates fully on-chain. Under this new model, staking pool managers, who assess and price risk using different methods and frameworks, determine the minimum price they are willing to accept for a given risk. Staking pool managers don’t need to be whitelisted to launch a public pool, but it is advised that all possess sufficient risk expertise and have the capacity to effectively increase distribution. Multiple staking pools can underwrite the same risk but price it differently and offer different amounts of capacity via NXM staking. When a user gets a quote in the UI, they are directed to the pool with the lowest price that has enough capacity for their request. A new “Multi-router” update will allow coverage capacity to be sourced from multiple pools. However, in the event of a claim payout, each pool would only be exposed to the amount of capacity sourced from their respective pool. This ensures that risks remain compartmentalised and manageable, providing a balanced approach to risk sharing and coverage.
The main difference between V1 and V2 is that NXM staking only determines capacity, not price. The pricing adjusts to changes in demand and utilisation, allowing a staking pool to diversify risk across cover products. If utilisation is low, then the price will start to decrease toward the minimum price set up by the manager. If there's a sudden rush to buy cover, then the price will increase quickly in a short period of time, so the mutual can control supply and demand via pricing and capture more revenue when exposure to risk changes considerably in a short period of time. This allows staking pools to diversify risk across cover products, and pricing to be reactive to market conditions without action from any staking pool managers.
Additionally, surge pricing is applied to cover products when capacity falls between 90% and 100% reserved for existing covers. This new pricing model provides users with a more accurate representation of risk and makes it easier for them to obtain coverage at a fair price (in line with the laws of demand and supply).
InsurAce
The InsurAce Protocol team introduced a new pricing model based on the belief that staking-driven pricing structures fail to accurately assess a protocol's risks and result in cover providers charging too much for coverage when fewer funds are staked. To address this issue, they developed a dynamic price model that sets a minimum and maximum premium. The base premium is determined by a combination of the aggregate loss distribution model, commonly used in traditional insurance, and the risk factors associated with the protocol itself. This model uses historical data to train two separate models, the frequency model and the severity model, to calibrate the probability of a given number of losses occurring during a specific period and to produce the distribution of loss amounts. The resulting aggregate loss is then incorporated into protocol risk factors, and calculations for the base price of each protocol are formulated.
However, given the difficulties in gathering historical on-chain data and the fact that their pricing structure was off-chain, concerns arose regarding transparency and the potential for price manipulation.
InsurAce pricing model has undergone a transformation, with the team adopting different off-chain pricing models, that are not publicly disclosed anymore. The pricing models vary depending on the product, and use risk scores and historical data to calculate the premium price and determine the capacity offered as a percentage of the TVL.
Ease
Ease, introduced the RCA model, and technically, reciprocally-covered assets do not require a detailed risk assessment to function. Since no premiums are charged for coverage, Ease is able to cover protocols without a specific risk assessment, with the Ease DAO's initial approval or denial of the protocol following a rigorous investigation by the entire community serving as the figurative risk assessment. When an exploit event occurs, the protocols that are deemed safer will get slashed less, whereas the least secure protocols will be slashed the most. It ultimately relies on the same premise as Nexus Mutual V1, namely that the community is accountable for performing due diligence on projects and assessing their risk through Ease token delegation, since the vaults that enjoy more Ease token delegation will get slashed the least, while the vaults that have the least token delegation will be slashed the most.
To ensure the stability of the ecosystem, the DAO has assigned the vaults in Ease a predetermined capacity. This prevents an individual vault from becoming excessively large compared to others. Therefore, a scenario where a single protocol has an interest in safeguarding a substantial TVL covered by Ease cannot occur. The system is designed to prevent any vault from overshadowing others with its contents, maintaining balance and diversity in the ecosystem is crucial.
The advantage of this system is that risk is distributed across the entire ecosystem rather than being carried by a single vault or protocol, and users are not required to pay premiums unless an exploit occurs. Given that the risk is shared by all users and vaults, users are not genuinely insured in the conventional sense. Rather, they do not lose all of their capital in the event of an exploit, only a portion.
Since risk is proportionally distributed among users, a larger hack will result in larger payouts to users, but will never lead to complete insolvency, resulting in a much more resilient coverage model. Additionally, the user's funds are never fully covered, as there is a capacity restriction on the vaults in order to maintain solvency. As an illustrative example to explain the mechanisms, let's consider the unlikely scenario where 25% of the RCA ecosystem is simultaneously hacked. In such a scenario, only 75% of the stolen vaults would be reimbursed, as impacted vaults will only be compensated an amount equal to the losses of other vaults, assuming a simultaneous and equal loss across all vaults. In another example, where a certain vault experiences a 25% loss in TVL, this loss will be distributed among the entire ecosystem. To estimate the reimbursement in this last example, one would need to consider the number of other vaults and calculate the approximate slashing of each vault, taking into account the distribution of gvEase delegation.
The Ease team attempts to prevent this by refraining from introducing new protocols to the ecosystem, conducting thorough audits of existing and future protocols, and performing diligent evaluations of protocols they consider adding.
Unslashed
Unslashed introduced a pay-as-you-go policy that allows users to terminate the cover at any time, with payments being calculated live. Pricing depends on several factors: besides a fair pricing methodology applied to each policy or policy type, Unslashed considers the correlations between policies that belong to the same bucket. The pricing also takes into account loss distributions, as it does in traditional actuarial pricing.
The most recent policies include a supply and demand curve, allowing the premium to vary with the utilisation ratio. The team states they have onboarded quants from traditional finance and managed to build and calibrate models that allow Unslashed to fairly price risk and structure insurance products. However, none of these models are public, and as such, they bear natural intrinsic risks, i.e., trust is required. There is no information on how the calculation is performed, how weights are assigned to each factor, or whether this is a behind-closed-doors process reviewed by the team or accepts input from governance.
Considering that Unslashed uses a pay-as-you go model, this is most likely run off-chain due to the costs of doing this on-chain. Another insurance protocol, Armor (later Ease), implemented an on-chain pay-as-you-go policy, but had this discontinued as Ethereum fees rendered it unsustainable.
NSure
Nsure also introduced a dynamic pricing model based on supply and demand to determine policy premiums. Their model employs the 95th percentile of a beta distribution (Beta(α, β)), and the shape parameters are capital demand and supply.
The team recognises that due to the lack of historical data on smart contract exploits, it is difficult to apply traditional actuarial pricing to Nsure products. They argue that for transparency's sake, it is beneficial to use a supply-and-demand model that is easily verifiable. This means that the more insured value there is in DeFi and, in particular, in Nsure, the less sensitive premium pricing will be to demand and supply changes, which increases the robustness of the insurance landscape. However, in the case of Nsure, the less the price is driven by supply and demand, the more it would be influenced by a risk cost that is currently determined by the team in a non-transparent way, which could be problematic.
The premium is also influenced by a risk factor that accounts for the project's level of security and a cost loading that accounts for claim settlement costs and other internal expenses. Without this factor the premium rate of two projects would be the same if their capital demand and supply were the same, which is not ideal. Nsure developed the Nsure Smart Contract Overall Security Score (N-SCOSS), a 0 to 100 rating system for determining the risk cost for every project based on five major characteristics: History and Team, Exposure, Audit, Code Quality, and Developer Community. The NSURE team assigns a weight to each category and performs due diligence on each project by rating each category. However, finding a decentralised way to assess this risk factor would be an improvement.
The team points to some improvements that could be made to the system, such as introducing an adjustment variable to credit for strengthening or penalising something that may not have been captured within the 5-pillar structure. Another future improvement mentioned by the team refers to the data sources, since Nsure has been using sources such as SlowMist Hack Zone, DeBank and DefiPulse, but wants to set up an automatic data feed into the rating model via external data aggregation, minimising manual interference. This could minimise centralised judgement and, in the future, make N-SCOSS an auto-generated indicator for users' reference. This concern to make Nsure risk assessment more transparent, unbiased, and available for all is definitely a step in the right direction. Another potential improvement would be for new factors to be added through governance, as well as the corresponding weights. However, it’s worth mentioning that there have been no updates about NSure since early 2022.
Risk Harbor
For Risk Harbor, the pricing is automatically determined by the AMM, which takes market conditions and protocol risk into account.
When underwriters deposit funds into the pool, they pick a Price Point at which they are willing to assume risk. The Price Point is the proportion of the overall underwriting amount a potential user will pay in advance when buying protection from the protocol. These premiums would flow to the underwriters who had deposited funds at the chosen Price Point.
Users searching for coverage monitor the available pricing points and purchase at any Price Point with sufficient unused underwriting capital. If the consumer desires more coverage than the one available at a single price point, they can split their order across multiple price points.
The price depends on a variety of things. First among these are the assessed hazards of the protocol for which protection is being sold. Risk Harbor’s team decides how to weigh those hazards before feeding them to the AMM. The second factor to evaluate is the amount of outstanding protection that has been sold. Risk-averse, the protocol prefers to spread its liabilities. This means that if protection on a certain pool is in great demand, the AMM will propose a higher price for protection on that pool. This works in a similar way to dynamic pricing based on demand and supply. Likewise, if the protocol feels it bears commitments that are connected with the protection you are attempting to purchase, the price will be higher because the protocol is risk-averse.
A risk-on vault, for instance, indicates that the vault is not particularly risk-averse. Risk-on vaults are appropriate for underwriters with a high risk tolerance, such as large, diversified hedge funds. A risk-off or conservative vault is preferable for underwriters with a reduced risk tolerance, such as DAOs and pension funds.
The risk model is one of the inputs for cover pricing. The risk cost is expected to follow the probability distribution of default occurrences, informing the AMM of the likelihood of a default event occurring in each of the vault’s pools. The risk model also includes the correlation between different occurrences, as in the case of Nsure. However, no information could be found regarding how the default occurrence probability needed for the risk cost is obtained, how the risk cost is integrated into the cover pricing, or if it’s done on-chain or off-chain, so a more in-depth analysis is not possible.
Bridge Mutual
Like InsurAce, Bridge Mutual uses a dynamic price model based on the utilisation ratio, i.e., the supply and demand of a cover. The considered variables are the utilisation ratio of the pool, the duration of the cover, and the amount covered. As each of these goes up, the price of coverage also goes up. Bridge Mutual establishes a minimum (1.8%) and maximum (30%) premium. An utilisation ratio above 85% is considered risky for the protocol, and as such, the pricing of the premium increases more rapidly.
The risk cost for Bridge Mutual is the utilisation ratio. A high utilisation ratio implies that many users are willing to take insurance against the project, but few are ready to provide coverage, hence, the project is considered risky. However, these pools charge higher premiums and hence have a higher APY, which can drive the utilisation ratio down by attracting more underwriters. There is no other direct evaluation of risk other than the utilisation ratio, which cannot always be a correct measure of risk.
To ensure there is enough liquidity in a pool to pay all outstanding covers, coverage providers are forced to wait 4 days before withdrawing their USDT after a withdrawal request. They can only withdraw up to the amount that pushes the utilisation ratio of the particular coverage pool to 100%. Withdrawals are also only possible when there are no active claims against them. This can potentially create a poor user experience for projects with small coverage pools, and it could perhaps be mitigated by an incentive structure with a focus on these smaller pools. Without this concern for proper incentivization, it is difficult for users to take advantage of their ability to create new coverage pools for uncovered protocols.
Sherlock
Sherlock, is an auditing firm where protocols are the ones being insured, not users. The price is based on a fixed percentage of the TVL and caps it based on the maximum amount of coverage that Sherlock can offer. The cover premium was fixed at 2% for each protocol that completes a public audit, and fixed at 2.25% for private audits, but the team increased the prices to 3.0% and 3.5%, respectively. To ensure that a protocol does not overpay for coverage, the monthly premium is updated based on an off-chain script that manages the TVL being covered that month.
In order to stay current on payment, the protocol must keep the balance above a minimum USDC amount (currently 500 USDC) and a minimum amount of seconds of coverage left (fixed to 12 hours). If a protocol's active balance drops below either of these thresholds, an arbitrager can remove the protocol from coverage for a fee.
Sherlock usually requires a month or so of upfront payments before coverage can be activated. From there, it's up to the protocol team in terms of paying bi-weekly, monthly etc. All Sherlock monitors is that the balance of payment does not reach zero.
Given that code audits require significant time, expertise, resources, and manpower, one of Sherlock's challenges was scalability, as Sherlock is only able to expand as more protocols are covered, which requires more code audits prior to providing that coverage. To combat this, Sherlock recently announced a new code audit contest initiative, through which code auditors can compete to provide audits to Sherlock for DApps (also known as Watsons) that they wish to underwrite.
Sherlock's theoretical foundation is based on the low probability that multiple maximum payout events will occur within a short time span and drain the capital pool, leaving protocols without coverage. An objective quantitative risk analysis could give more security to this foundation. If a large payout reduces the capital pool by 50%, there will still be sufficient capital in the pool to cover the same amount of coverage for another protocol. Sherlock's clients value the coverage even though they are aware that the likelihood of other protocols draining the capital pool is extremely low. While this skin-in-the-game approach reveals confidence in the audits done, in the eventuality of a large exploit occurring, Sherlock's entire value proposition may be put at risk. Sherlock's code audits could, by proxy, lack the same trustworthiness, which could cause stakeholder funds to be removed from the capital pool, lowering the TVL, and effectively diminishing Sherlock's ability to cover more protocols in the future due to a lack of funds.
Neptune Mutual
The Neptune Mutual uses a dynamic pricing model based on various factors such as the type of contract, the contract's risk score, and the amount of coverage being purchased to determine the premium for coverage.
The pricing model works by first assessing the risk score of the contract, which is calculated based on various parameters such as the contract's complexity, the size of the contract, and the value at risk. Once the risk score has been calculated, it is used to determine the base premium rate for coverage. As the names suggest, the base premium is the lowest possible cover price that occurs when only a small amount of the underwriting liquidity of the pool has been utilized; which encourages users to purchase policies.
The purchase policy then incorporates various factors, such as the amount of coverage being purchased and the current demand for coverage on the platform, to determine the final premium rate. As the utilisation ratio increases towards 100%, the price of cover policies increases; this encourages users to provide liquidity to the cover pool because liquidity providers returns are generated by the cover fees paid by policy purchasers.
Cozy Finance
Cozy Finance introduced On-chain Programmatic Pricing in their recent V2 release. With this new functionality, market creators gain the flexibility to utilise pre-existing cost templates or design their own pricing models. These on-chain pricing models offer a high level of customization, enabling market creators to tailor their models to meet specific requirements.
However, it's important for market creators to be mindful of the gas fees associated with these complex pricing models. The execution of complex on-chain pricing models can incur higher gas costs compared to simpler models. Therefore, market creators should consider the trade-off between model complexity and gas fees when designing their pricing strategies.
By empowering market creators with the ability to deploy and customise pricing models on-chain, Cozy Finance V2 opens up new possibilities for creating dynamic and innovative markets. It encourages experimentation and creativity while urging market creators to be mindful of the gas costs associated with their pricing models.
Comparing Pricing Models
The table below offers a comprehensive overview of the pricing models utilised in the industry, distinguishing between those employed on-chain and off-chain. Each model has its own unique advantages and considerations, including the accuracy of risk assessment, alignment with demand and supply dynamics, and fairness in pricing.
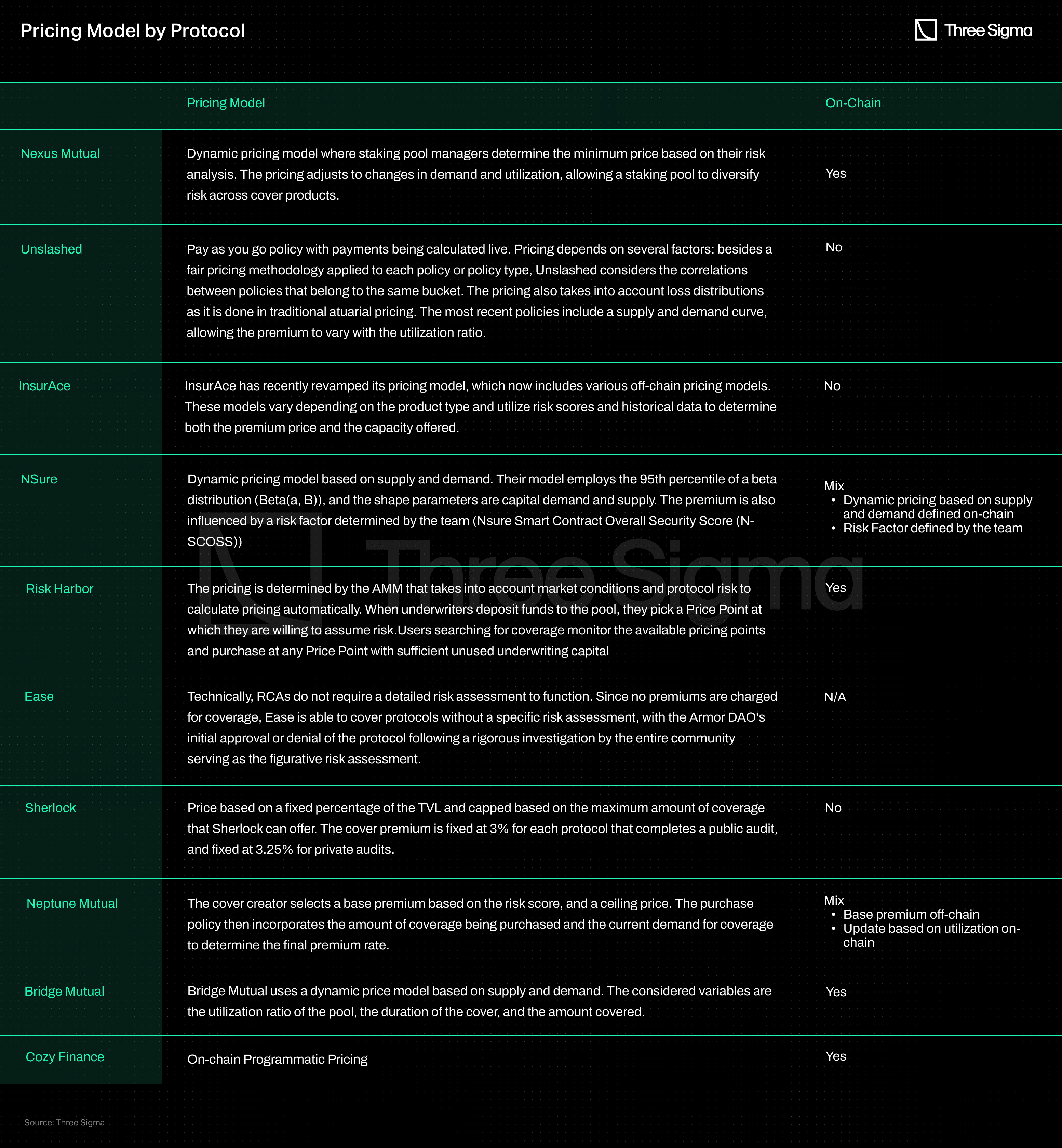
By considering the relationships between different risk factors, premium rates can also be adjusted based on the level of interdependence and potential contagion between risks. Correlation allows for a more holistic evaluation of risk factors, enhancing the effectiveness and accuracy of risk assessment.
Correlating risk in DeFi insurance models becomes even more critical due to the composability and lego-like development of services built on top of each other or with strong dependencies. The interconnected nature of protocols and their dependencies highlights the need for accurately assessing the correlations between risks.
Without proper risk correlation, the impact of events in one protocol or pool on others may be overlooked. Currently, DeFi insurance risk models often address this challenge by creating separate pools for each protocol or coverage. However, this approach limits the ability to comprehensively assess and manage the overall risk landscape. By accurately assessing and addressing interdependencies, insurance protocols can better understand and manage risks, ensuring the long-term viability and resilience of the market.
Final Thoughts
DeFi insurance protocols face several limitations when it comes to pricing and risk mechanisms that need to be addressed to foster a thriving ecosystem. These challenges include the demand for fixed premiums and market limitations on both sides of the market.
Initially, a market-based pricing mechanism was employed, combining risk calculations with the capital locked up by cover providers to determine premiums. However, this approach assumed that cover providers would stake more funds in safer protocols. The influence of expected APY on capital providers' incentives might cloud their risk assessment judgement, leading to potential inaccuracies in reflecting a protocol's risk. Furthermore, many users lack the technical expertise to conduct proper risk assessments, further complicating the situation. Therefore, the value staked may not accurately reflect a protocol's risk.
Another constraint is the limitation of both sides of the market. Insurance protocols that cap the amount of coverage available suppress the demand for insurance, resulting in lower annual percentage returns (APRs) for LPs and making underwriting in DeFi insurance less attractive.
To overcome these challenges, an insurance protocol should incorporate supply and demand mechanics. By allowing the cost of coverage to increase during times of high demand, the protocol can balance the market.
While implementing prediction markets or derivatives may not be feasible due to capital efficiency and the need for counterparties, it is crucial to design the market to minimise the impact of protocol flaws. By addressing these challenges, the DeFi insurance market can become more appealing to underwriters, leading to a stronger and more resilient ecosystem. Ultimately, the objective is to create a market that incentivizes sophisticated players to identify under- or overpriced risks, driving the market towards efficient pricing and robust risk management.
The next article will dive into existing claim assessment processes being implemented in the DeFi Insurance space.
Methodology:
To conduct our analysis, we first utilised the Defillama Insurance Category List, which provided us with a comprehensive overview of insurance protocols operating on the Ethereum ecosystem. We then reviewed each protocol on the list, excluding those that did not meet our criteria for analysis. The exclusion criteria included the halting of operations, changing focus, or unavailability of publicly accessible information:
- Cover Protocol suffered a hack in 2020 and was shut down in 2021.
- Armor.Fi decided to introduce the RCA Coverage model and rebranded to Ease.org in May 2022.
- iTrust Finance had no publicly available documentation.
- The UnoRe documentation did not fit with what the protocol is currently offering.
- The InsureDAO team is currently working on revamping the protocol, and there is no public information on pricing mechanisms other than it happens off-chain.
- The Solace team is currently working on revamping the protocol, and there is no public information on pricing mechanisms.
- Tidal Finance has no public information on its pricing model.
- ArCx, Ante Finance, and Helmet are not insurance protocols. ArCx is an analytics platform; Ante Finance is building a trust rating; and Helmet is an options protocol that can be used to hedge exposure, but it is not an insurance protocol by design.
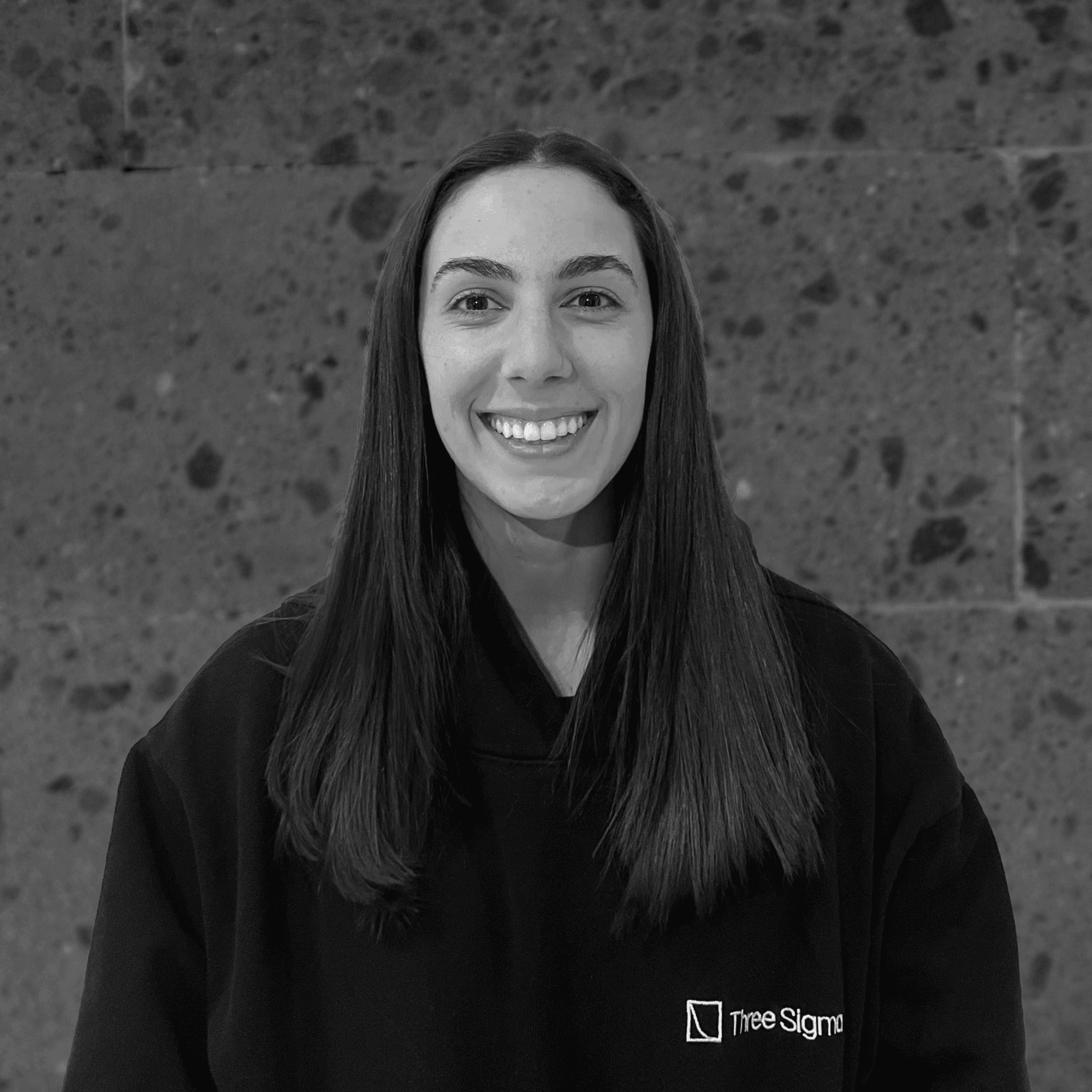
Catarina Urgueira
Researcher
Catarina has a Master’s in computer science and is a seasoned professional with a strong background in blockchain technology. As a former blockchain team manager at Vodafone and current team lead of Three Sigma’s Capital department, she brings valuable experience and expertise in both leading teams and understanding the technical aspects of blockchain.